The American Statistical Association is launching a public relations campaign to make people think statistics is less boring and pointless, which is good:
We want students and parents to have a better understanding of a field that is often unknown or misunderstood. Statistics is not just a collection of numbers or formulas. It’s not just lines, bars or points on a graph. It’s not just computing. Statistics is so much more. It’s an exciting—even fun—way of looking at the world and gaining insights through a scientific approach that rewards creative thinking.
That’s a quote from the shiny new website, ThisIsStatistics. It has stories about what statisticians do, and information about salary and job trends and stuff. There are videos of statisticians talking about their work: currently Roger Peng (Johns Hopkins, SimplyStatistics blog) and Genevra Allen (Rice University).
It’s slightly disappointing that more of the people on the site arent’ real, just stock photos, but I suppose that’s unavoidable. What’s a bit more annoying is one of the photos in particular:
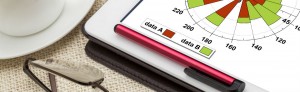
This looks as if it was constructed specially (the cup/mat/tablet/glasses are stock, eg). It’s a rose chart, which is an ok way to display circular data (eg wind directions), but is not so good for comparison because of the way the wedges change shape as they get larger. The numeric labels are also a slightly strange choice for a circle measured in degrees (90 isn’t a multiple of 20).
Much more importantly, given the emphasis of the site on statistics as solving real problems, this is labelled as not being real: “data A” and “data B”. Not helpful when we’re trying to tell people “It’s not just lines, bars or points on a graph”.